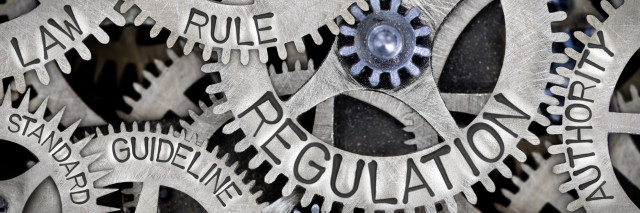
Commentary on NAIC's Casualty Actuarial and Statistical Task Force White Paper - "Regulatory Review of Predictive Models"
It likely would not come as a news flash to anyone reading this blog that insurers have continued to increase their use of predictive analytics, technology and data to improve their ability to select and price risk and streamline their operations. It’s hard to attend a trade show or read a news feed that doesn’t talk about the value that investments in such aspects can provide.
For good reason. Increased use of data and analytics can help insurers avoid adverse selection, better identify and serve customer needs and reduce their cost structure by using machine learning algorithms or robotic process automation (RPA), which can perform certain tasks that used to require human intervention. This allows an insurer to focus their human capital on tasks that machines are not so good at, including providing empathetic customer service.
However, while predictive analytics can provide significant benefits to insurance companies and customers, the rapid pace at which analytics is evolving and the relative complexity of some of the models used poses a significant challenge to state regulators who are charged with reviewing and approving such models.
The National Association of Insurance Commissioners (NAIC) recognized this emerging issue and created the Casualty Actuarial and Statistical Task Force (CASTF), which has been charged with identifying best practices to guide state insurance departments in their review of predictive models for underlying rating plans.
Over the course of the last year, the CASTF has released multiple drafts of the white paper “Regulatory Review of Predictive Models” for public comment. And comment the public has! Numerous letters have been submitted from trade associations, actuarial organizations, credit agencies, consumer groups and even insurance departments to provide their input on the lengthy white paper.
While the paper has taken a few different forms across versions, the stated purpose is to provide best practices that “promote a comprehensive and coordinated review of predictive models across states” and adhere to the following principles1:
- State insurance regulators will maintain their current rate regulatory authority.
- State insurance regulators will be able to share information to aid companies in getting insurance products to market more quickly.
- State insurance regulators will share expertise and discuss technical issues regarding predictive models.
- State insurance regulators will maintain confidentiality, where appropriate, regarding predictive models.
The most significant challenge with an undertaking like this, in my opinion, is to provide sufficient guidance to cover many modeling situations across jurisdictions of varying insurance department staffing—while not being overly prescriptive and onerous. Doing so would fly in the face of some of stated principles such as promoting speed-to-market.
In addition, it would not seem to be in the overall public good if such a regulatory framework served to stifle innovation in the insurance industry. (In fact, these are not unlike some of the challenges encountered with the recently released Actuarial Standard of Practice No. 56 on Modeling.) To try to address some of these challenges, as currently drafted the paper focuses on the very common Generalized Linear Model (GLMs), and states that the best practices contained within are to merely provide guidance to a regulator and not serve as a checklist. That said, the paper literally contains about 75 items that a regulator should consider obtaining during review of a predictive model.
Categories of such items include:
- the data used to build the model;
- adjustments made to the data;
- predictor variables used;
- model validation processes and performance measures;
- comparison of old model to new model; and,
- how the model is accurately translated into the rating plan.
Some of the specific items requested seem benign (provide a listing of input variables to the model and their source), but some items are much more concerning (explain the “rational connection” for each variable to impact on the target variable). Further, these best practices are assigned an importance level from one to four to help the regulator determine which of these many items are most relevant. The issue that could arise is that the items in the paper are taken as a blanket checklist or cause misunderstanding between the regulator and filer of the model.
It is not clear at the of time of this writing what the final version of the white paper will look like, or when such a final version will be issued as discussions are ongoing. The general premise of capturing such best practices is a good one. The challenge will lie in the execution. And, regardless whether or not such a paper is issued or how it is used, it stands to reason that insurance departments are already starting to take their cues from these drafts in terms of types and amounts of information to request of insurers when it comes to filing predictive models.
Now, more than ever, it is critical for an insurer to put sufficient forethought into how a model will be used and how it will be received by stakeholders (including regulators). Strong documentation throughout the model building process will also serve an insurer well. Finally, being proactive with regulators and having discussions about potentially challenging modeling situations sooner rather than later is key.
Pinnacle Actuarial Resources has decades of experience building and implementing predictive models. In this ever-evolving and complicated environment, we’d welcome the opportunity to discuss your next predictive modeling project to help you most effectively realize your goals.
1. https://content.naic.org/sites/default/files/call_materials/White%20Paper%20%26%20Comments.pdf