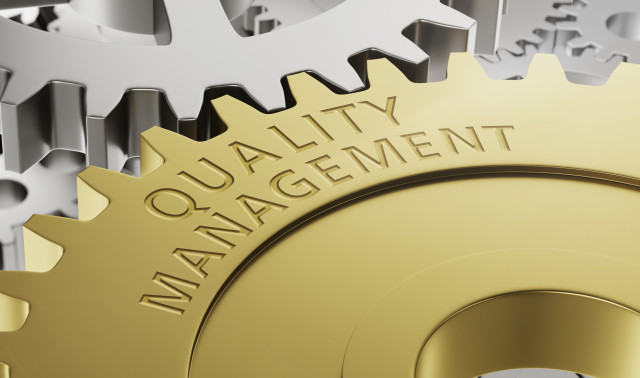
The Importance of Data Management and Data Quality
On the May 2020 Pinnacle APEX Webinar, “The Importance of Data Management and Data Quality,” we addressed a critical business issue: the costs of poor data, reasons for data management, and ways to improve how data is managed in an organization. The webinar also took a deep dive into methods to address the major challenge of missing data.
Data is the foundation to any analytics project. The value of analytics work can be critically undermined if the data is poor. Even the best actuary or data scientist is hard-pressed to overcome issues with bad data.
A 2016 study from IBM estimated the annual cost of poor data quality in the United States at $3.1 trillion. For context, this is the combined market capitalization of Facebook, Amazon and Apple, Inc. That estimated annual cost of poor data quality comes from sources including reduced employee productivity and sub-optimal analytics results. A recent Gartner study estimated that the average annual financial impact of poor data quality per organization is $9.7 - $14.2 million, or a whopping 30% of revenue.
Further, it is estimated that globally 82% of organizations are operating without an optimized strategy for data management. In the insurance industry it varies slightly by type of insurance company, but it is estimated that approximately 27% of employees’ time is spent on data quality issues while about one-third of projects are adversely impacted by poor data quality.
In addition to the costs in both time and resources of poor data quality, actuarial professional and regulatory considerations support the need for a strong data management program and data quality practices.
For example, the American Academy of Actuaries’ Actuarial Standard of Practice 23—Data Quality (ASOP 23), addresses steps an actuary should take when performing actuarial services. Some of these steps include disclosing significant limitations of the data being used and assessing if data is appropriate, sufficiently current and reasonable for the project.
Notably, ASOP 23 does not require that an actuary perform an audit on the data, which further supports the need for a solid data management program. In addition, the National Association of Insurance Commissioners (NAIC) Casualty Actuarial and Statistical Task Force (CASTF) has now issued two versions of a draft document, with a third expected soon, called “Regulatory Review of Predictive Models.” The document is intended to provide guidance and best practices to state insurance departments as they review predictive models in rate filings.
The best practices for data quality can be organized into three different categories: establishing a data management program, data quality for data stores and data quality for datasets. There are several ways to enhance data quality in each category in turn.
We discussed five key points of focus within each of these three categories:
- Establishing a Data Management Program
- Getting support at the top levels of the company
- Sharing the word about the importance of data management broadly across an organization
- Developing a solid data governance framework
- Assessing the current state of the quality of an organization’s data environment
- Investing in metadata
- Continued Quality of Data Stores
- Clearly defining allowed values and performing periodic audits of the data
- Automating data entry and balancing data to established sources
- Periodically refreshing data
- Having strict change controls and a well-documented change process
- Preparing data stores for data privacy compliance
- Data Quality for Datasets
- Assessing reasonableness of data and comparing to other sources and time periods
- Looking for outliers in the data
- Incorporating subject matter expertise and peer review into the process
- Using data visualization techniques to review the dataset
- Documenting data adjustments
As part of our webinar, we conducted a review of missing data issues and discussed methods for handling them. Missing values are generally the most common issue with “dirty” data, and can affect the accuracy of most machine learning algorithms. Even small amounts of missing data in a variable can add up to a significant problem when viewed across all variables in a dataset.
Missing values in data also can create bias and reduce the power of the model being developed. While there are many ways to address the challenge of missing data, some are more recommended than others given the impact they have on the results. In short, however, simply ignoring missing values (arguably the easiest solution) does not make the problem go away and generally is not recommended.
Some of the common methods for addressing missing data such as dropping variables, listwise/pairwise deletion, using the mean/median/mode for imputation, or even applying a simple regression framework can undermine the quality of the data and result in lesser performing models. Instead, we recommended that more advanced methods including multiple imputation, maximum likelihood and generative adversarial imputation networks (GAINs) as they provide for less bias and much more robust model results.
In conclusion, there are many reasons for an organization to devote effort to improving their data quality practices, including high costs of poor data quality, lost productivity, and other, various professional and regulatory considerations. However, it is clear that data quality is not a spectator sport. It takes hard work, resources, planning and dedication by an organization to get it right. While data is seen as the fuel that drives the engine, it is only quality data that will really get an organization where they need to be.
Pinnacle thanks all of those who joined our APEX Webinar: “The Importance of Data Management and Data Quality.” Let us know how we can help develop ways to enhance your data quality and help your business.