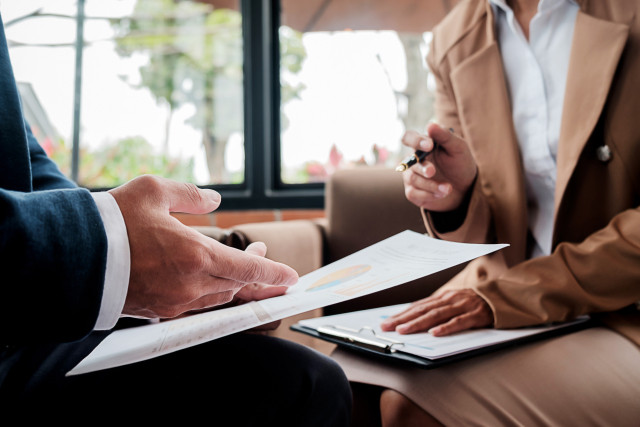
The Modeling Lifecycle - Don't Break the Chain!
When it comes to advanced analytics such as building predictive models, many people immediately think about the vast amounts of data, computing horsepower needed, and very sophisticated (often mysterious) techniques applied to the data to produce the results. Without question, all of these aspects are important steps in the process. However, there are several other critical steps both before and after these that can truly make or break an advanced analytics project. We can think of this as the Modeling Lifecycle.
Importantly, when it comes to the Modeling Lifecycle, we should also think about it as a truly cyclical process, as illustrated below. While there can be different flavors of the Modeling Lifecycle, a good way to think about it includes the following steps.
- Defining the business question or problem to be solved (Business Question)
- Determining the data needed and data available (Data)
- Identifying the methods to be applied in constructing the model (Methods)
- Exploring the data (EDAs)
- Iterating on steps of variable reduction and model building (Model Building)
- Validation of the model/quality checks (Model Validation)
- Implementation of the model (Model Implementation)
- Monitoring of the model and reporting of results (Model Monitoring)
There are, of course, several important sub-steps included in those above. But at a high level, this provides a view of the general lifecycle involved in the development of a model. Even if a predictive model is not the chosen methodological approach for a given business problem, many of these same kinds of steps will apply.
In today’s world, successful companies across industries, and especially in the insurance industry, are more data driven in their decision making than ever before. We should expect this trend to grow, with data serving as the raw material to fuel that growth. Taking that raw material and being able to transform it into a useful format to inform those decisions though is the essential aspect.
What likely jumps out from the previous picture is that all of these steps are connected along the way—and—they cycle back to the beginning (the defining or understanding of the business issue to be tackled). This is a key point of the entire process. The predictive model is being built not for the fun of it, but to address a particular business question or problem. The model is to be (part of) the solution. Naturally as well, when something is implemented, it must be monitored to see if that something is accomplishing the results that were intended. If so—great! If not, then the lifecycle must be re-entered to determine what steps along the way need to be reworked.
What aligns to all of this is the importance of clear communication and understanding of the industry and business challenges facing our clients today. This is critical not only at the onset of the project but also throughout the entire lifecycle. Being able to consistently execute this process—transforming the raw material of data into valuable business insights—is imperative to creating a data driven culture within an organization. This is especially essential for an insurance company to not just survive but thrive. Without this, an insurance company runs the risk of being data-rich yet decision-poor, which is not the recipe for success. Pinnacle brings both the technical expertise to address a business problem utilizing advanced analytics as well as the ability to clearly communicate with our clients throughout. It’s that focus on clarity of communication that helps set Pinnacle apart.