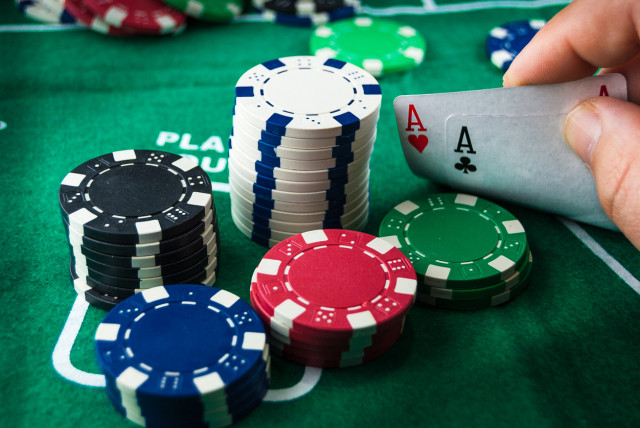
"To Some Arbitrary N, and Beyond!"
Pinnacle University (Pinnacle U) is an annual industry event put on by Pinnacle Actuarial Resources. It’s a collaborative event where actuarial science students from various university programs partner with our actuarial analysts to research and present compelling topics in the insurance industry.
We were very pleased to be chosen to participate in the Pinnacle U program for 2020. Pinnacle U is usually an in-person event, but this year has brought some unique challenges to our society. For the first time, Pinnacle U was presented virtually rather than as a traditional, in-person event. This shift was due to work from home restrictions created by state government in response to the Coronavirus pandemic. It took a lot of coordination by everyone involved to present Pinnacle U and our many thanks to all those so involved with the coordination of the event.
We decided to investigate the ever-evolving role of simulation in insurance, with a focus on the practicality and implementation of the Monte Carlo Simulation (MCS). Our presentation followed a funnel approach starting with the general procedure for any type of simulation. We then narrowed in on theoretical and practical methods and uses of simulations for the insurance industry. Finally, we focused specifically on MCS and a few applications of that particular simulation technique.
Developed, in part, by Stanislaw Ulam in the late 1940s, MCS is a robust method of parameter estimation that relies heavily on sampling to make inferences or predictions about population values. In practice, a practitioner, such as a statistician or an actuary, would start by making assumptions about underlying data distributions, simulate values from these distributions a number of times and then assess or interpret outcomes. In our presentation, we demonstrated the power and flexibility of MCS through a few practical examples constructed in RStudio.
In our first example, we explored estimating percentage of losses eliminated via a deductible by simulating varying claim amounts from a lognormal distribution. Our expectation was that the indicated deductible factors would converge at some number of iterations. That expectation was indeed realized at approximately 10,000 to 25,000 simulations. When analyzing parameter convergence, however, we noticed something that initially seemed a bit odd—our sample variance failed to converge for several hundred thousand samples. Digging further, we found the explanation in the essence of what makes MCS function: the law of large numbers.
The law of large numbers asserts that as the number of samples increase, the sample average will converge to its true value. This principle has no mention of the sample variance. Upon closer inspection of the general formula for variance listed below, we notice that the numerator sums the squared distances from the mean. The operative word here is ‘squared’ which translates to a high degree of weight being placed on outliers. It takes a much larger sample size to smooth the effect of outliers on the variance. This insight enhances the conclusions of our next example, where we saw just how many samples are required to equalize the effects of variance.
Our second example surveyed an aggregate loss distribution. Basic R functions were used to simulate a number of claims from a frequency distribution and individual loss amounts from a severity distribution. Losses were modified using a policy limit. We ran the simulations several times to obtain large samples of simulated total losses to be used for further statistical analysis.
Simulations using slightly different severity distributions produced notably different simulated samples. We concluded that care should be exercised in distribution selection before constructing any simulation. Our examination of confidence intervals around the mean showed that only a moderate number of simulations—approximately 10,000—were needed to estimate the mean with a high degree of confidence. An analysis of the 99th percentile showed that it would need more than 100,000 simulations to estimate the additional 1% or the tail of the distribution with a high level of confidence.
We found that MCS is a flexible and powerful tool for estimating losses, and modeling insurance products. This is because simulation techniques allow for the generation of large samples and can be used to estimate parameters with a high degree of certainty—due to the law of large numbers.
Specifically for insurance applications, we saw that estimates of the mean converge with a moderate number of simulations. That insight can help facilitate informed decision-making about average losses. By contrast, estimation of the tail requires more simulations, but may be necessary for reinsurance or determining risk-appetite. We are eager to see how MCS will continue to penetrate the predictive modeling domain as automation and reliance on big data becomes more and more prevalent.
For more information about Monte Carlo Simulation, please follow the link below:
Steve Jagodzinski is an Actuarial Analyst with Pinnacle Actuarial Resources, Inc. in the Bloomington, Illinois office. He holds a Bachelor of Science degree in actuarial science from Illinois State University. Steve has experience in assignments involving Loss Reserving, Group Captives and Loss Cost Projections. He is actively pursuing membership in the Casualty Actuarial Society (CAS) through the examination process.
Taylor Daigle is an Actuarial Analyst with Pinnacle Actuarial Resources, Inc. in the Bloomington, Illinois office. He holds a Bachelor of Science degree in mathematics with an actuarial science concentration from Louisiana State University. Taylor has experience in assignments involving Loss Reserving, Group Captives and Predictive Analytics. He is actively pursuing membership in the CAS through the examination process.
Mani Venkateswaran is a current graduate student of the Actuarial Science Program at Illinois State University. He holds a Bachelor of Science degree in mathematics from Rensselaer Polytechnic Institute. Mani has experience and completed coursework related to Financial Mathematics, Engineering, Probability Theory and Applications, Statistical Methods, and Simulation Theory. He is actively pursuing membership in the CAS through the examination process.